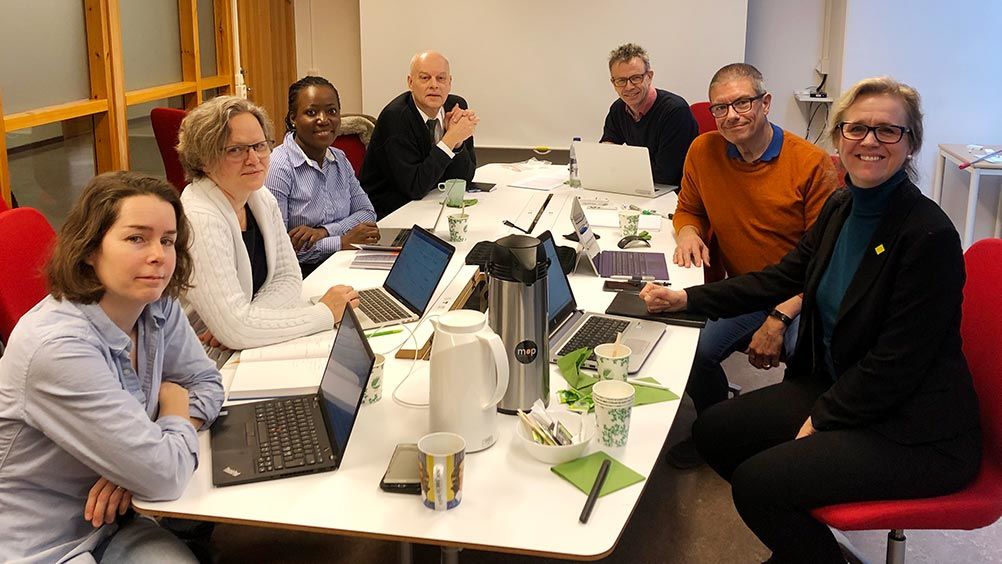
ESS will have Sweden's most complex control system and uses machine learning to optimise operational reliability. Through several collaborations with industry and technology companies in machine learning and AI, ESS also contributes to digitalisation and technology transfer in industry.
ESS is basically an extremely advanced and complex machine, designed and built to provide neutron beams for research that are brighter than those from any other neutron source existing today. However, to be able to attain high goals of operational availability and prevent downtime, ESS makes the use of machine learning – an application within artificial intelligence (AI) that enables complex systems to automatically learn and improve from experience without being explicitly programmed.
Accelerator-based research facilities such as ESS are among the world’s most complex systems, encompassing tens of thousands of devices that are monitored and managed by intricate control systems which integrates the operations of all facility infrastructures through a network of hardware, software and configuration databases.
An important toolbox to enhance the control system functionality at ESS
The Integrated Control System (ICS) at ESS plays a key role for the machine performance and operational reliability of the facility, and manages about 1.6 million control signals from 100,000 devices in a large variety of systems, both advanced and non-advanced. Machine learning will therefore be an important toolbox to enhance the control system functionality at ESS.
“In 2019 we made a strategic decision to launch a Control System Machine Learning project at ESS, to ensure making the best use of machine learning and AI for the facility operation,” says Head of Integrated Control System, Henrik Carling. “We hope to increase facility efficiency by utilising modern machine learning technologies in combination with the control system.”
The Control System Machine Learning (CSML) Project within the ICS Division focuses on how AI and machine learning can be applied to the control system in order to increase the operational efficiency at ESS, while reducing operational and maintenance costs as well as decreasing commissioning time and effort. The project dedicates a full-time position, for an initial period of four years, to exploring machine learning aspects for implementation in the control system.
“It is very exciting to be involved from the start in this work, which will be key for ESS and involve more of us over time,” says Karin Rathsman, Accelerator Physicist and Project Manager for the CSML project at ESS. ”Two years into the CSML project we are confident that AI, in particular machine learning, will change the way we operate Big Science facilities and industrial processes.”
Helping advance machine performance and functionality
Machine learning is becoming increasingly important for all large research infrastructures with complex control systems, in order to advance machine performance and functionality. As large-scale research facilities build on scientific collaborations and are used to working with open data and open software, cooperation on machine learning applications is a natural step. Last autumn, ESS and the synchrotron facility DESY in Hamburg, Germany, initiated a long-term collaboration within this field, by arranging a joint workshop, focusing on failure detection and prevention.
Potential catalyst for Industry 4.0
Research facilities such as ESS have access to large amounts of control system data that can be used to drive development in AI and machine learning, to the benefit of industry and academia. Today’s control systems in industry are less complex and have far fewer control signals, but they are becoming increasingly advanced as a result of the on-going digitalisation in industry, the so-called 4th Industrial Revolution. Collaboration with industry is also beneficial for ESS, as shared industrial standards and processes can be implemented in the facility’s control system.
In a recent pilot study, ESS joined forces with the Faculty of Computer Science at Lund University (LU), Big Science Sweden (Sweden’s Industrial Liaison Office) and the tech company GoalArt to explore how data from the ESS control system can be used externally for research and innovation in AI and machine learning.
The pilot study, ESS Control System Data Lab, was coordinated by Lund University and funded by the Swedish Innovation Agency, Vinnova, the Wallenberg AI, Autonomous Systems and Software Program (WASP), and the Region of Skåne. As alarm management is a key element in all complex systems, both within research facilities and industry, this initial study focused on alarm data. A reference group, with representatives from industry (such as ABB, Tetra Pak and Hitachi), Big Science facilities (DESY) and academia (LU) could confirm the potential value of collaboration around ESS control system data, enabling advanced R&D within machine learning.
“We conclude in our report that by sharing data, ESS can function as a catalyst for Industry 4.0 digitalisation, both in industry and other research facilities,” explains LU’s Per Runeson, Professor Software Engineering. "Data sharing fulfills the function of sharing knowledge, and our project shows that it is possible for ESS to be a role model and share relevant data with industry," he adds.
In another recent machine learning project funded by Vinnova, ESS has been collaborating with the tech company DVel to develop machine learning models that can predict issues during operation, before components break or the process stops, and implement these algorithms in the control system.
”The knowledge transfer from ESS and other research facilities are of great importance for the technological development and digitalisation in Swedish and European industry,” says Anna Hall, Director Big Science Sweden. “The collaboration projects within machine learning are one example of how ESS contributes to innovation and increased competitiveness, for SMEs as well as large companies.”